Weighing the Effectiveness of Photoplethysmography Signal Analysis in Population Diagnosis of Atrial Fibrillation
Review paper by Miles Henderson, biomedical student at the Amsterdam University College.
Photoplethysmography has become an accessible component of smartwatches in recent years. The primary advantage of these devices is that they provide a semi-accurate and continuous estimate of the user’s heart metrics. Similarly, PPG technology has also been explored as a tool for the large-scale detection of arrythmia in the populace. Atrial fibrillation is the most common arrythmia, is difficult to detect, and often remains asymptomatic. Moreover, the current gold-standard diagnostic for atrial fibrillation relies on electrocardiographic ambulatory monitoring to generate data for detection. This paper argues that this technology may be limited in its effectiveness and its high costs represents a non-trivial obstacle to large-scale deployment. As a more inexpensive and compact technology, PPG provides a unique solution to the detection of asymptomatic atrial fibrillation in the populace. This paper evaluates existing algorithmic techniques for the autonomous detection of atrial fibrillation using PPG data, which have been iterated upon in recent years. New approaches that substantially improve classification performance are assessed, and obstacles to successful implementation are discussed.
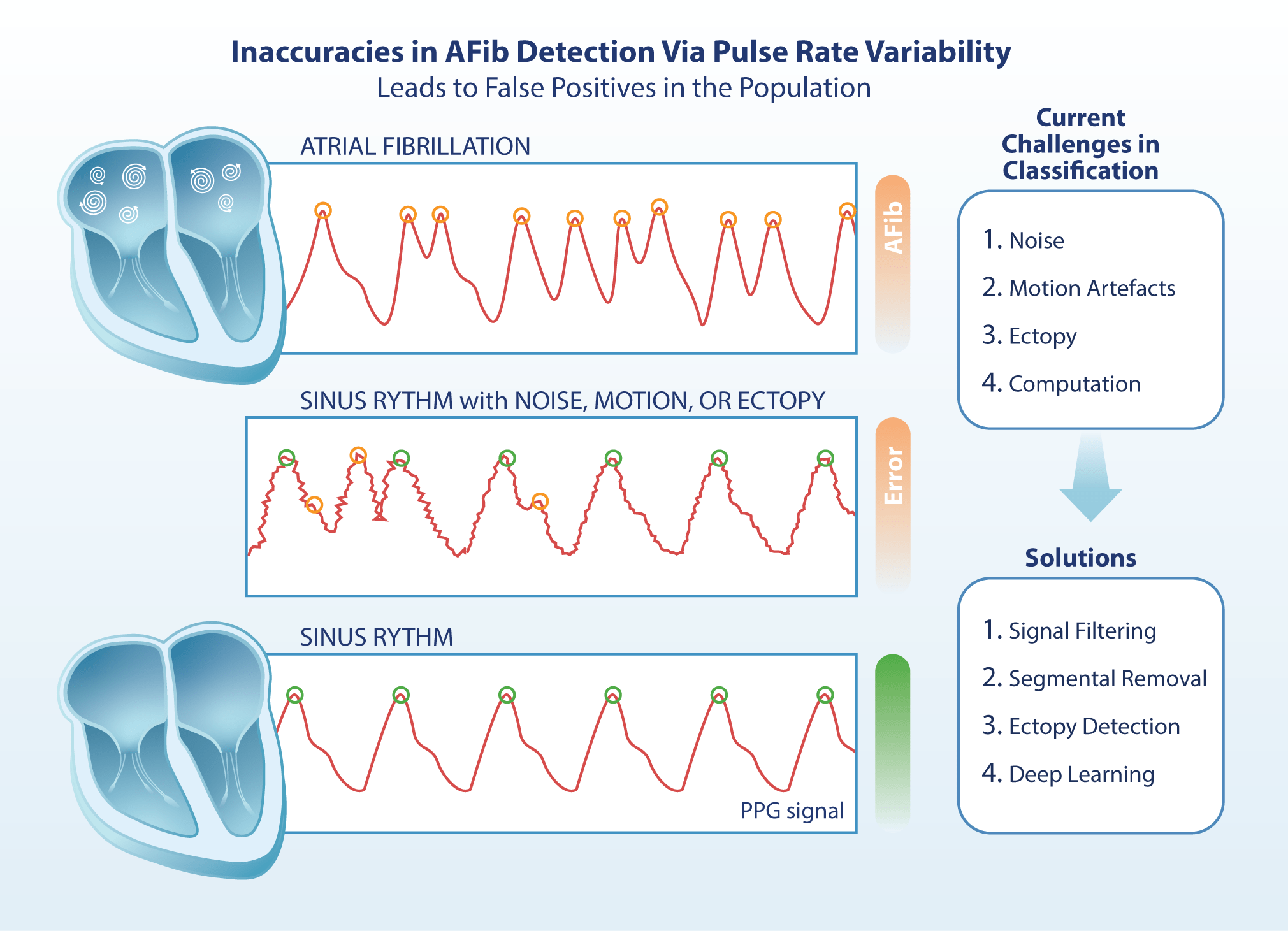
Introduction to Pulsatile photoplethysmography
Pulsatile photoplethysmography (PPG) is a well-established technique for quantifying the perfusion of peripheral tissues using reflected light; which, in recent years, has made it an economical tool for sampling heart rate in smartwatches. Since the introduction of PPG technology in consumer electronic devices, several studies on the clinical applications of remote health monitoring have been published (2, 4, 6, 9). In particular, the recently completed Apple Heart Study, with over 450 thousand participants, presents compelling evidence that algorithmically processed PPG signals obtained from consumer devices can detect Atrial Fibrillation (AFib) with some accuracy in the populace (11).
Affecting around 4.5 million diagnosed Europeans, AFib is the most common form of arrythmia and is a driver of cardiovascular events such as heart failure and ischaemic stroke (1, 12). Moreover, the healthcare costs associated with AFib are heavily attributed to patient care after admission; in Europe the economic burden of AFib is estimated to be between €660 to €3,286 million (12). Thus, early detection of AFib is crucial for early interventions and treatments that may improve long-term outcomes for patients and reduce healthcare costs (5).
One strategy that may reduce costs and enable large-scale detection of AFib is the utilisation of Photoplethysmography Waveform Variability (PRV) analysis. As a method of detection that is not restricted by short windows of data, high costs, or specialised medical assessment, PPG data can be processed into statistical indices for algorithmic detection (8).
Heart Rate Variability (HRV), on the other hand, requires R-R interval data from an ECG. Although HRV analysis has a high sensitivity for AFib, it can also be estimated using PRV derived from accessible PPG data (7). The greatest advantage to this substitution is that PRV does not require expensive nor invasive equipment, whilst being highly correlated with HRV (4).
As opposed to other non-invasive devices like the Holter monitor, the use of PRV sensors in AFib detection may be an economical and deployable alternative to the conventional ECG for large-scale diagnoses (5, 11). However, it is important to weigh the limitations of PPG sensors — which would be used in a non-stationary and non-clinical settings (4, 7). This means that the bulk of the challenge lies in the application of complex signal processing and AFib detection algorithms.
To assess the role of PRV indices in the future of large-scale AFib detection, this narrative review will assess its efficacy in early detection by evaluating current limitations in large-scale deployment. First, a contextual overview of difficulties in detecting AFib will be synthesised. Then, standard strategies and algorithmic approaches for AFib classification will be weighed in the context of large-scale detection. The limitations of this technology will also be discussed in the context of motion artefacts, ectopic beats, and sympathovagal balance. To conclude, this paper will weigh the extent to which PPG signal analysis is an effective large-scale strategy for AFib detection.
Difficulties in detection of Atrial Fibrillation
AFib is characterised by electrophysiological abnormalities that manifest as disordered high frequency activation of the atria (10). Its disruption of normal sinus rhythm and the variability of disordered contractions it causes means that it can be detected via a conventional electrocardiograph (ECG) (5, 10). However, the spontaneity of episodes in paroxysmal AFib (PAF), as well as the prevalence of asymptomatic AFib, make its detection with ECGs difficult (5). Notably, asymptomatic and paroxysmal AFib still confer a greater risk of ischaemic stroke (14).
The greatest complication in AFib detection is capturing short paroxysmal episodes, which can occur and disappear spontaneously (12). In fact, the majority of individuals with paroxysmal AFib do not transition to a more persistent and easily detectable form. As a result, these individuals may continue to evade detection (12). Adding to the obstacles in detection is the discovery that there is a very weak correlation between symptoms and AFib episodes. The 3-year LOOP study has shown, using implantable loop recorders in high-risk patients, that 90% of diagnosed individuals in its cohort were asymptomatic at the time of AFib detection (13).
To increase the odds of AFib detection in high-risk individuals, previously effective strategies have relied on expensive ambulatory ECGs that provide continuous data for 24 to 48 hours (5). This can present a significant cost. A 2015 study on the cost-effectiveness of monitoring AFib patients revealed that the cost of 24-hour Holter Monitoring to be as high as €471 per patient in Sweden, a country with a robust and inexpensive health care system (6). In addition to high costs, Ambulatory ECGs are also limiting in that they require more rigorous compliance from patients, requiring careful electrode placement, whilst ultimately carrying lower sensitivity than conventional ECGs (6, 7).
An alternative is the use of implantable loop recorders (ILRs), which have a minimum 3-year lifetime (13). Despite higher sensitivity and a much longer period of continuous data, ILRs present a challenge as they require invasive surgical implantation at non-trivial costs (5). Importantly, many ILRs with automatic AFib detection algorithmically process recorded R-R intervals using heart rate variability indices (5). This allows AFib episodes to be logged autonomously over long periods of time. Although a critical improvement over ambulatory ECGs, ILRs are limited by their need to be surgically implanted, as well as by their high cost. Given that the incidence of asymptomatic AFib is substantial within the populace, this strategy may pose a barrier to large-scale implementation.
For these reasons, a considerably more effective alternative may be non-invasive PPG technology. This could be in the form of an inexpensive wristband with a PPG sensor, a smartwatch, or other mass-produced consumer electronic devices such as smartphones. The advantage of PPG over conventional technologies is its capacity to be implemented on a large-scale at low cost whilst maintaining acceptable sensitivity. These processed signals could then be monitored algorithmically for AFib episodes, allowing for mass-diagnosis of asymptomatic AFib in the populace.
PRV and The PPG Signal to quantify the heart beat
HRV and PRV, broadly speaking, both quantify the variability of successive heart beats. They can be interpreted via similar parameters, which provide information about the variability of consecutive ventricular contractions. AFib manifests as irregular atrial depolarisation, which propagates to the ventricles through the atrioventricular node, leading to correspondingly irregular ventricular contractions. This can be distinguished from normal sinus rhythm by analysing specific intervals in a PPG or ECG signal. However, there are differences in the type and quality of intervals analysed. HRV derives its intervals from R-R peaks on an ECG, which are the result of electric depolarisation of the ventricles. These intervals represent the quasi-periodic phases of the cardiac cycle (4, 8). PRV, on the other hand, derives its intervals from either local minima or maxima, which are substitutes for R-R peaks. This is important to note as the inherent susceptibility of PPG technology to movement and interference means that noisy PPG data must be processed before local extrema can be accurately identified (15).
Noise Attenuation and Signal Pre-processing. The main objective of signal processing is to prepare the signal for the identification of important signal features, whilst avoiding false identification. Although this paper focuses on two digital filters, it is pertinent to note that multiple techniques can be applied to process digital data. These range from the removal of corrupted signal segments, to transformations into the frequency domain for further analysis (15). Although outside of the scope of this paper, frequency domain analysis may be less susceptible to motion disturbances and can be used in conjunction with time-domain indices like PRV (7, 15).
Before PRV can be calculated, however, it is common practice to filter the signal (15). This serves to isolate signal frequencies to a desired physiological range, or bandpass, which reduces the noise that may result from motion (figure 1). For example, the gold-standard Butterworth filter could be applied on a lowpass (<5Hz) to isolate any signal under 300bpm. This is extremely important for large-scale implementation, as these sensors would be subjected to sustained and inconsistent activity.
The order and type of a filter are also highly relevant. Whilst higher-order filters attenuate the transition between passband and stopband, they are also more computationally intensive. In a multi-filter comparison to find the optimum filter
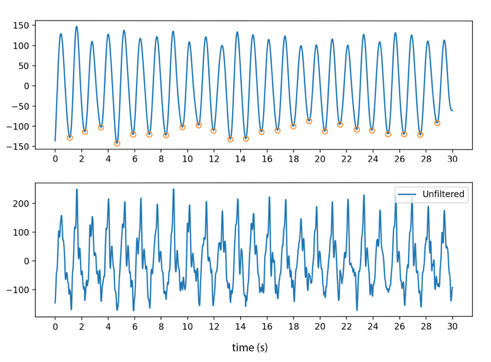
Figure 1: The lower graph depicts a 30-second raw noisy PPG signal, with standardised amplitude on the y-axis. The graph above depicts a processed noisy signal that allows local extrema to be distinguished. This signal was empirically processed and evaluated for this paper using data from an open dataset (20).
Noise Attenuation and Signal Pre-processing
The main objective of signal processing is to prepare the signal for the identification of important signal features, whilst avoiding false identification. Although this paper focuses on two digital filters, it is pertinent to note that multiple techniques can be applied to process digital data. These range from the removal of corrupted signal segments, to transformations into the frequency domain for further analysis (15). Although outside of the scope of this paper, frequency domain analysis may be less susceptible to motion disturbances and can be used in conjunction with time-domain indices like PRV (7, 15).
Before PRV can be calculated, however, it is common practice to filter the signal (15). This serves to isolate signal frequencies to a desired physiological range, or bandpass, which reduces the noise that may result from motion (figure 1). For example, the gold-standard Butterworth filter could be applied on a lowpass (<5Hz) to isolate any signal under 300bpm. This is extremely important for large-scale implementation, as these sensors would be subjected to sustained and inconsistent activity.
The order and type of a filter are also highly relevant. Whilst higher-order filters attenuate the transition between passband and stopband, they are also more computationally intensive. In a multi-filter comparison to find the optimum filter for PPG processing, a 4th order Chebyshev II filter produced the highest quality signals compared to the gold-standard Butterworth filter (24). Liang et al. identified this filter based on inferred signal quality from signal symmetry. Interestingly, both Liang et al. and Elgendi et al. emphasise that the Chebyshev II filter produced a more distinguished dicrotic notch — an artefact of isovolumetric relaxation, when there is a slight increase in blood pressure due to aortic valve closure (24, 25). However, in the context of AFib detection the presence of salient dicrotic notches may lead to the false detection of local extrema within the signal (figure 2). When extrema detection algorithms are employed, this can cause erroneous feature identification, increasing the chances of false detection.
This poses a unique problem in the context of large-scale detection. A small rate of false positives will consume unnecessary additional resources, possibly negating the benefit of large-scale PPG detection over conventional ambulatory monitors. For example, the recently completed Apple Heart Study demonstrated that in participants where an irregular pulse was detected, 84% of the algorithmically logged episodes were consistent with AFib. Even less (34%) were confirmed to have AFib in follow-up ambulatory monitoring (16).
Feature Identification
The next conventional step after time series data is cleaned, detrended and denoised by filters is to algorithmically identify local extrema. In a single cardiac cycle, the PPG waveform exhibits three quasi-periodic features that can be consistently identified: peak systolic perfusion, the dicrotic notch, and start of systole (figure 2). For purposes of beat identification in AFib classification, several papers use either peak systolic perfusion (local maxima) or start of systole (local minima) (15, 18). Unlike the sharp R peaks of an ECG, which result rapid depolarisation, the peaks and troughs on a PPG waveform are more rounded. It has been proposed that this could introduce small inaccuracies in feature identification (26).
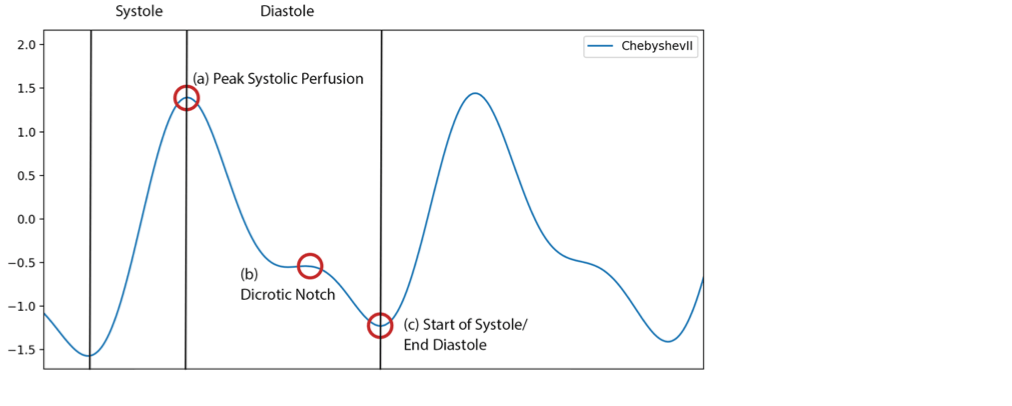
Figure 2: A filtered signal, 4th order Chebyshev II. Three quasi-periodic features are indicated. Start of Systole approximates the R peak on an ECG; however, Peak Systolic Perfusion can also be used. Periods of the cardiac cycle are delineated by vertical lines. This signal was empirically processed and evaluated for this paper using data from an open dataset (20).
Classification using PRV
The prevailing consensus is that the irregular atrial activation characteristic of AFib is non-deterministic and random (22). Multiple papers have tried to quantify this property via different indices for AFib classification (22). While some indices capture an estimate of signal complexity, such as Shannon Entropy, PRV quantifies beat-to-beat variability (Table 1). In particular, we will discuss the root mean square of successive interval differences (RMSSD), which is a standard PRV index. Several approaches that utilise RMSSD as an index for the algorithmic detection of AFib have been implemented (4, 15, 17, 18). These are usually in the form of an RMSSD threshold. Interestingly, when RMSSD is estimated with PPG data and compared to its equivalent in HRV, there is an extremely high correlation (r > 0.99) with no statistically significant difference (19). Moreover, it has shown that R-R intervals themselves are almost perfectly correlated with PPG peak intervals (26). This means that RMSSD calculated from PPG intervals can serve as an effective alternative for ECG.
To this end, it was a seminal paper in 2009 that first demonstrated RMSSD could be used to algorithmically detect AFib episodes in real-time by using ambulatory ECG data (18). A more recent paper on the effectiveness of 314 algorithms in the estimation of respiratory rate has also revealed that the 100 highest performing algorithms include a time-domain component like RMSSD (16).
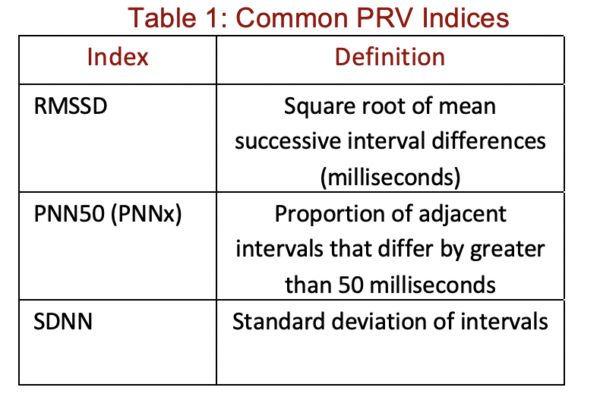
Ectopic Beats
Unlike AFib, premature ventricular contractions are not pathological and can occur sporadically throughout normal sinus rhythm. However, this present a problem in large-scale detection. Multiple papers have determined that the classification accuracy of interval-based algorithms can drop when ectopic beats occur (15, 18, 22). In a recent paper comparing multiple PRV indices, RMSSD performed poorly in classification of AFib against ectopy (accuracy: from 81% to 54%) (22). This has led to application of several strategies to counter this effect. For example, Bashar et al. revealed that when an ectopic beat detection algorithm is applied to PPG data, detection performance increases (15). In their example, accuracy increased significantly from 95.54% to 98.09%.
Nevertheless, if an algorithm is implemented on a large-scale, even a small rate of misclassification on ectopic beats could lead to a non-trivial number of false positives.
Sympathovagal Balance
Heart rate is regulated by the autonomic nervous system (ANS) via a balance in sympathovagal stimulation of the heart. Sympathovagal balance is altered under several unique conditions that are well documented, such as orthostatic hypotension and respiratory sinus arrythmia. In addition to motion artefacts in the PPG signal, these phenomena can also affect variability. In the context of non-stationary conditions, time-domain indices like RMSSD are highly subject to these changes in autonomic regulation. For example, whilst RMSSD from PRV is highly correlated with its HRV equivalent (r = 0.998) in the supine position, this correlation can drop somewhat (r = 0.970) as a result of a change in posture (26).
Weighing PRV Against Other Approaches
Commonly used time-domain indices like RMSSD are used in combination with frequency-domain or non-linear indices to improve classification performance. However, Lee et al. first demonstrated that a RMSSD threshold on PPG data, alone, could correctly detect AFib with strikingly high accuracy, sensitivity, and specificity: 98.44%, 97.63%, and 99.61% respectively (17). Important to note is that the experimental protocol collected data from patients in the supine position, without introducing motion.
More recent research indicates that once motion is introduced, the accuracy of this approach to AFib classification decreases substantially, with one experiment showing that AFib classification on a noisy signal can have a false positive rate of 53.74% (15). Several techniques have previously been applied to try to attenuate the inaccuracies introduced by motion. These range from pre-processing via filters, as previously discussed, to adding non-linear indices like Sample Entropy to the algorithm. One successful strategy has been to identify corrupted signal segments and remove them. In one application of this approach, Bashar et al. demonstrated that by using accelerometer data to detect and erase high motion segments, false positives could be brought down by 22.22% (Table 2) (15).
The growing body of artificial intelligence-based techniques have also been applied to AFib classification. Unlike existing approaches that require high-quality data, a deep learning approach can maintain high performances without removing corrupted segments, thereby drastically improving signal coverage (9). Importantly, compared to an RMSSD-based approach, a convolutional neural network demonstrated a much higher area under the ROC curve (AUC) despite higher signal coverage and a much higher degree of noise. Some important caveats are that this approach still suffers somewhat from the misclassification of ectopic beats, and that this approach is computationally intensive.
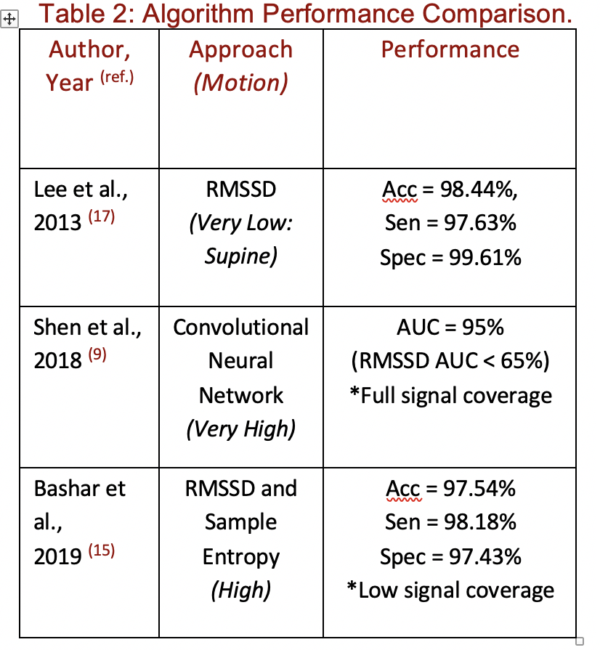
Discussion and Future Directions
PRV is a tried and true approach to quantitatively classify irregular heart activity. Applied to the PPG signal as a threshold, standard indices like RMSSD have proven to produce impressive classification accuracies that approach 100%. With increasingly large datasets and greater access to health metrics, PPG signal analysis presents a unique opportunity to diagnose asymptomatic AFib in the populace. Not only would this allow newly diagnosed patients to undergo early treatments and interventions, potentially extending quality of life, this may also reduce the large healthcare burden of AFib.
Nevertheless, false positives are one of the biggest limitations to the large-scale implementation of PPG signal analysis. Although many approaches to AFib classification are accurate in low-motion settings, once PPG sensors are introduced to environments with high degrees of noise, classification accuracy drops considerably. The variation in sympathovagal balance, as well as the lowered classification accuracy caused by ectopic beats also compound this problem.
Current statistical strategies to attenuate misclassification rely heavily on pre-processing, and more recently on the deletion of corrupted segments or ectopic beats to ensure high-quality input. Encouragingly, new artificial intelligence-based techniques have achieved impressive signal coverage and classification performances without removing confounding segments. Nonetheless, almost all algorithms appear to suffer from the occurrence of ectopic beats. Moreover, some of the more complex techniques, that benefit from a nominal increase in accuracy under these conditions, would also be more computationally intensive. This is the main drawback of high-performing approaches like convolutional neural networks. It is also an especially relevant point for the application of PPG signal analysis to user owned devices, where computational power comes at the expense of portability.
Despite the previously outlined obstacles that lie in the way of successful implementation, recently completed large-scale studies like the Apple Heart Study have made PPG technology a promising avenue for continued AFib research — establishing that a non-trivial degree of classification accuracy can be achieved in the populace at low cost. Ultimately, future research is needed to iterate on existing strategies for AFib detection, whilst providing solutions to persistent problems such as ectopy, noise, and excessive computational requirements.
The AFIP team congratulates Miles Henderson with his excellent thesis on ‘PPG in AF detection’ and wishes Miles good luck with his study and future directions.
References
- Chugh SS, Havmoeller R, Narayanan K, Singh D, Rienstra M, Benjamin EJ, et al. Worldwide Epidemiology of Atrial Fibrillation. Circulation. 2014;129(8):837–47.
- Gambi E, Agostinelli A, Belli A, Burattini L, Cippitelli E, Fioretti S, et al. Heart Rate Detection Using Microsoft Kinect: Validation and Comparison to Wearable Devices. Sensors. 2017Feb;17(8):1776.
- Graham IM. The importance of total cardiovascular risk assessment in clinical practice. European Journal of General Practice. 2006;12(4):148–55.
- Jong G-J, Aripriharta, Horng G-J. The PPG Physiological Signal for Heart Rate Variability Analysis. Wireless Personal Communications. 2017Aug;97(4):5229–76.
- Keach JW, Bradley SM, Turakhia MP, Maddox TM. Early detection of occult atrial fibrillation and stroke prevention. Heart. 2015Feb;101(14):1097–102.
- Levin L-A, Husberg M, Sobocinski PD, Kull VF, Friberg L, Rosenqvist M, et al. A cost-effectiveness analysis of screening for silent atrial fibrillation after ischaemic stroke. Europace. 2014;17(2):207–14.
- Shaffer F, Ginsberg JP. An Overview of Heart Rate Variability Metrics and Norms. Frontiers in Public Health. 2017;5.
- Sassi R, Cerutti S, Lombardi F, Malik M, Huikuri HV, Peng C-K, et al. Advances in heart rate variability signal analysis: joint position statement by the e-Cardiology ESC Working Group and the European Heart Rhythm Association co-endorsed by the Asia Pacific Heart Rhythm Society. Europace. 2015;17(9):1341–53.
- Shen Y, Voisin M, Aliamiri A, Avati A, Hannun A, Ng A. Ambulatory Atrial Fibrillation Monitoring Using Wearable Photoplethysmography with Deep Learning. Proceedings of the 25th ACM SIGKDD International Conference on Knowledge Discovery & Data Mining – KDD 19. 2019;
- Staerk L, Sherer JA, Ko D, Benjamin EJ, Helm RH. Atrial Fibrillation: Epidemiology, Pathophysiology, and Clinical Outcomes. Circulation Research. 2017;120(9):1501–17.
- Turakhia MP, Desai M, Hedlin H, Rajmane A, Talati N, Ferris T, et al. Rationale and design of a large-scale, app-based study to identify cardiac arrhythmias using a smartwatch: The Apple Heart Study. American Heart Journal. 2019;207:66–75.
- Velleca M, Costa G, Goldstein L, Bishara M, Lee MB. A Review of the Burden of Atrial Fibrillation: Understanding the Impact of the New Millennium Epidemic across Europe. EMJ Cardiology. 2019Oct;7(1):98–109.
- Diederichsen SZ, Haugan KJ, Brandes A, Lanng MB, Graff C, Krieger D, et al. Natural History of Subclinical Atrial Fibrillation Detected by Implanted Loop Recorders. Journal of the American College of Cardiology. 2019;74(22):2771–81.
- Mahajan R, Perera T, Elliott AD, Twomey DJ, Kumar S, Munwar DA, et al. Subclinical device-detected atrial fibrillation and stroke risk: a systematic review and meta-analysis. European Heart Journal. 2018Oct;39(16):1407–15.
- Bashar SK, Han D, Hajeb-Mohammadalipour S, Ding E, Whitcomb C, Mcmanus DD, et al. Atrial Fibrillation Detection from Wrist Photoplethysmography Signals Using Smartwatches. Scientific Reports. 2019;9(1).
- Charlton PH, Bonnici T, Tarassenko L, Clifton DA, Beale R, Watkinson PJ. An assessment of algorithms to estimate respiratory rate from the electrocardiogram and photoplethysmogram. Physiological Measurement. 2016;37(4):610–26.
- Lee J, Reyes BA, Mcmanus DD, Mathias O, Chon KH. Atrial fibrillation detection using a smart phone. 2012 Annual International Conference of the IEEE Engineering in Medicine and Biology Society. 2012;
- Dash S, Chon KH, Lu S, Raeder EA. Automatic Real Time Detection of Atrial Fibrillation. Annals of Biomedical Engineering. 2009;37(9):1701–9.
- Lu S, Zhao H, Ju K, Shin K, Lee M, Shelley K, et al. Can Photoplethysmography Variability Serve as an Alternative Approach to Obtain Heart Rate Variability Information? Journal of Clinical Monitoring and Computing. 2007Jul;22(1):23–9.
- Liang Y, Chen Z, Liu G, Elgendi M. A new, short-recorded photoplethysmogram dataset for blood pressure monitoring in China. Scientific Data. 2018;5(1).
- Aliamiri A, Shen Y. Deep learning based atrial fibrillation detection using wearable photoplethysmography sensor. 2018 IEEE EMBS International Conference on Biomedical & Health Informatics (BHI). 2018;
- Fallet S, Lemay M, Renevey P, Leupi C, Pruvot E, Vesin J-M. Can one detect atrial fibrillation using a wrist-type photoplethysmographic device? Medical & Biological Engineering & Computing. 2018;57(2):477–87.
- Stein KM, Walden J, Lippman N, Lerman BB. Ventricular response in atrial fibrillation: random or deterministic? American Journal of Physiology-Heart and Circulatory Physiology. 1999Jan;277(2).
- Liang Y, Elgendi M, Chen Z, Ward R. An optimal filter for short photoplethysmogram signals. Scientific Data. 2018Jan;5(1).
- Elgendi M, Fletcher R, Liang Y, Howard N, Lovell NH, Abbott D, et al. The use of photoplethysmography for assessing hypertension. npj Digital Medicine. 2019;2(1).
- Gil E, Orini M, Bailón R, Vergara JM, Mainardi L, Laguna P. Photoplethysmography pulse rate variability as a surrogate measurement of heart rate variability during non-stationary conditions. Physiological Measurement. 2010;31(9):1271–90.
Do you know someone who might be interested in this?